Blend cuts AI inference cost by 85% on Salad while running 3X more scale
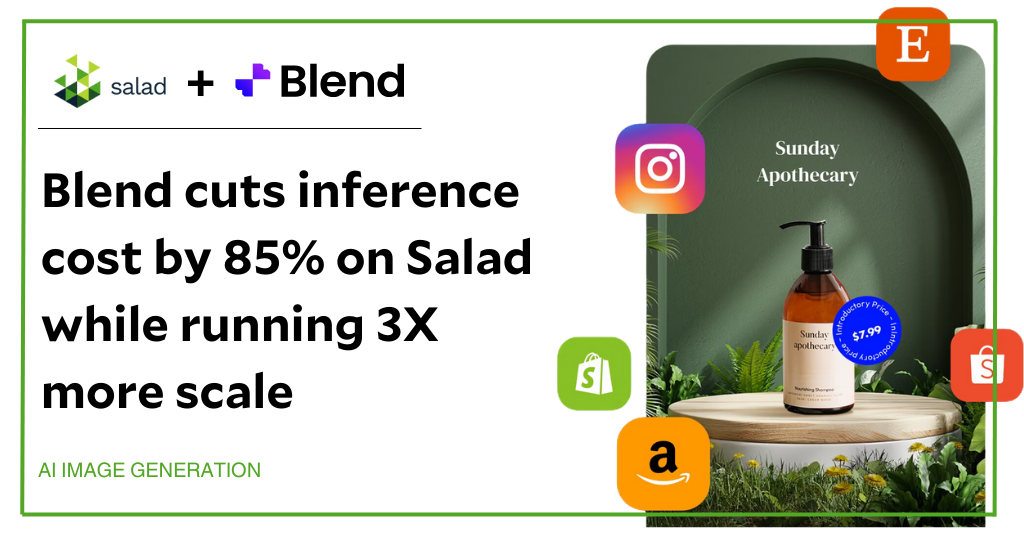
Startups often have a unique origin story. Blend is no different. Their origins lie in a Whatsapp channel and a local fireworks seller who printed 2000 physical posters for a peculiar reason. Blend is an AI copilot for e-commerce that helps sellers create professional product photos and designs in two clicks without hiring an agency. Their mission is to help entrepreneurs & small sellers grow sales online with compelling social graphics, product photos and SEO optimized copy. Today, Blend serves thousands of sellers generating around 6000 images every hour on Salad’s distributed network. In this chat with Jamsheed Kamardeen, Chief Technology Officer (CTO) at Blend, we discuss their growth, the switch to a distributed cloud, inference cost & more. How did the idea for Blend come about? It was during Covid-19. We were in many Whatsapp groups looking for common problems faced by e-commerce sellers and we found a peculiar thing. There were many coaching sessions on how to use photo and design apps. Turns out, many of the sellers didn’t have a design team on their own but they needed to promote their products on social media, create posters, ads and the like. In fact, one of my cousins had a fireworks shop in a small village in India and 70% of his sales came from posters on Whatsapp & Instagram. He’d go to a local printing shop and use the designer there to create & print out 2000 posters just so he could get a soft copy of the design for ads/promotions. He didn’t even bother distributing the physical posters. So looking at the challenges of local sellers in promoting their products in a digital world – that’s where the idea came from. Photo editing and design apps have been out there for a long time, right? Weren’t they sufficient? Yes. There were a lot of apps & horizontal design tools which were good for designers. But the sellers aren’t designers. Plus there is the paradox of choice. Most tools had 100s of templates, colors, etc and needed a significant time commitment. Plus, often the sellers ended up with a design that looked terrible because they tweaked too much or too little. So we decided to create Blend to offload the design decision making. Just upload a picture of the product and tell us what you want the offer to be. We’ll remove the background, put the product in appropriate settings and deliver a design with text. Our goal was always to get them the final design in the fewest clicks possible. Today, you have Millions of downloads for your app. How crucial was the arrival of Generative AI in your user growth? Our initial version included background removal and adding in an appropriate background with some other features. But generative AI completely changed the game for us. For example, if a shoe store wants to do a 25% off Diwali promotion, all they had to do was upload the product photo and describe the offer and event. With Generative AI & Stable Diffusion models, we can identify it’s a shoe, have LLMs make the decision on what to paint & such, create an aesthetically pleasing urban background, automatically create appropriate text with the right color scheme and deliver the copy. All it takes is a couple of clicks. This is what led to our massive user growth. Today, 40% of our users are individual sellers, so we are introducing a separate web app for them as well. With big growth comes big cloud bills. That must have been the case for Blend as well. What infrastructure challenges did you face here? Right. Since we are an AI first design company, inference became our biggest cost factor. Plus, we needed powerful GPUs to power Diffusion models. Sourcing GPUs to keep up with surge in demand quickly became a nightmare. The existing providers didn’t have the right options for a company like us. AWS only had multi cluster A100s but there was no single cluster A100 option. GCP or Azure had them but they were expensive. So we started looking for alternatives. We found a local provider who offered A100s for a cheaper price. But that came with reliability & scalability issues. We didn’t always have enough GPUs during times of higher traffic. I started losing a lot of sleep over this GPU shortage. We’re a small team, so when the machines go down, my sleep goes away. So again, we were looking for an alternative. That’s when we found Salad. How has switching to SaladCloud impacted your cost and scaling? When we switched from the hyperscalers to A100s with a local provider, we didn’t really think the cost could go any lower. But switching to Salad was eye-opening. On Salad’s consumer GPUs, we are running 3X more scale at half the cost of A100s on our local provider and almost 85% less cost than the two major hyperscalers we were using before. Plus Salad is much more reliable. We’ve migrated all current and new workloads to Salad. I’m not losing sleep over scaling issues anymore. On Salad’s consumer GPUs, we are running 3X more scale at half the cost of A100s on our local provider and almost 85% less cost than the two major hyperscalers we were using before. I’m not losing sleep over scaling issues anymore. Jamsheed Kamardeen, Chief Technology Officer (CTO) at Blend As a CTO, making the switch to a distributed cloud is a huge decision. What was the decision making process? That’s a good question. I was very skeptical initially about the reliability of Salad. From a technical standpoint, my major question was this: Compared to data centers with reliable internet, how am I going to have reliable workloads on random people’s computers on a distributed cloud? We needed to implement some solutions to make reliability strong but it wasn’t as difficult as I initially perceived it to be. One thing that helped us was the engineering support offered by Salad which made
Civitai powers 10 Million AI images per day with Salad’s distributed cloud
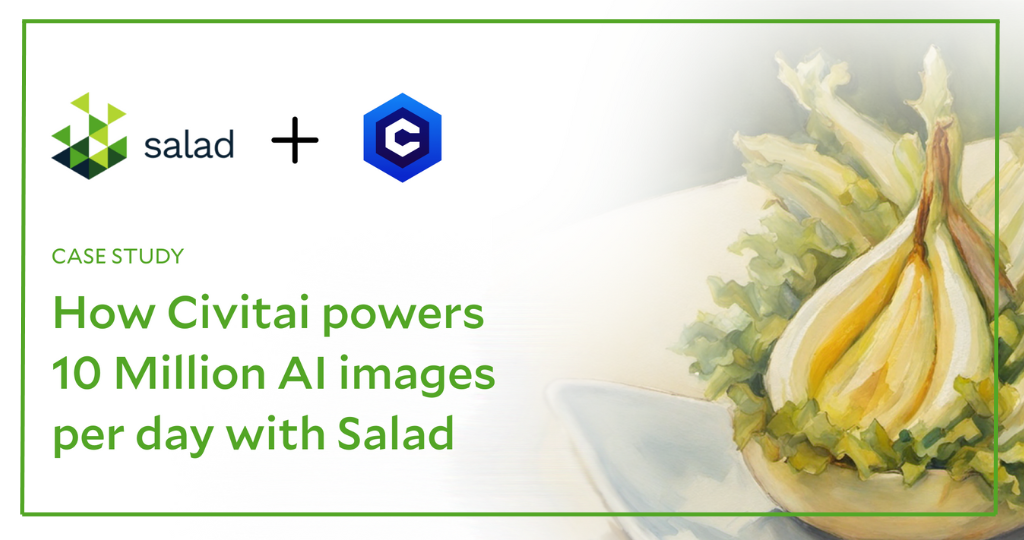
Civitai: The Home of Open-Source Generative AI “Our mission is rooted in the belief that AI resources should be accessible to all, not monopolized by a few” – Justin Maier, Founder & CEO of Civitiai. With an average of 26 Million visits per month, 10 Million users & more than 200,000 open-source models & embeddings, Civitai is definitely fulfilling their mission of making AI accessible to all.Launched in November 2022, Civitai is one of the largest generative AI communities in the world today helping users discover, create & share open-source, AI-generated media content easily. In Sep 2023, Civitai launched their Image Generator, a web-based interface for Stable Diffusion & one of the most used products on the platform today. This product allows users to input text prompts and receive image outputs. All the processing is handled by Civitai, requiring hundreds of Stable Diffusion appropriate GPUs on the cloud. Civitai’s challenge: Growing compute at scale without breaking the bank Civitai’s explosive growth, focus on GPU-hungry AI-generated media & the new image generator brought about big infrastructure challenges: Continuing with their current infrastructure provider and high-end GPUs would mean an exorbitant cloud bill, not to mention the scarcity of high-end GPUs. Democratized AI-media creation meets democratized computing on Salad To solve these challenges, Civitai partnered with Salad, a distributed cloud for AI/ML inference at scale. Like Civitai, Salad’s mission also lies in democratization – of cloud computing. SaladCloud is a fully people-powered cloud with 1 Million+ contributors on the network and 10K+ GPUs at any time. With over 100 Million consumer GPUs in the world lying unused for 18-22 hrs a day, Salad is on a mission to activate the largest pool of compute in the world for the lowest cost. Every day, thousands of voluntary contributors securely share compute resources with businesses like Civitai in exchange for rewards & gift cards. “For the past few months, Civitai has been at the forefront of Salad’s ambitious project, utilizing Salad’s distributed network of GPUs to power our on-site image generator. This partnership is more than just a technical alliance; it’s a testament to what we can achieve when we harness the power of community, democratization and shared goals”, says Chris Adler, Head of Partnerships at Civitai. Civitai’s partnership with Salad helped manage the scale & cost of their inference while supporting millions of users and model combinations on Salad’s unique distributed infrastructure. “By switching to Salad, Civitai is now serving inference on over 600 consumer GPUs to deliver 10 Million images per day and training more than 15,000 LoRAs per month” – Justin Maier, Civitai Scaling to hundreds of affordable GPUs on Salad Running Stable Diffusion at scale requires access to and effectively managing hundreds of GPUs, especially in the midst of a GPU-shortage. Also important is understanding the desired throughput to determine capacity needs and estimated operating cost for any infrastructure provider. As discussed in this blog, expensive, high-end GPUs like the A100 & H100 are perfect for training but when serving AI inference at scale for use cases like text-to-image, the cost-economics break down. You get better cost-performance on consumer-grade GPUs generating 4X-8X more images per dollar compared to AI-focused GPUs. With Salad’s network of thousands of Stable Diffusion compatible consumer GPUs, Civitai had access to the most cost effective GPUs, ready to keep up with the demands of its growing user base. Managing Hundreds of GPUs As Civitai’s Stable Diffusion deployment scaled, manually managing each individual instance wasn’t an option. Salad’s Solutions Team worked with Civitai to design an automated approach that can respond to changes in GPU demand and reduce the risk of human error. By leveraging our fully-managed container service, Civitai ensures that each and every instance of their application will run and perform consistently, providing a reliable, repeatable, and scalable environment for their production workloads. When demand changes, Civitai can simply scale up or down the number of replicas using the portal or our public API, further automating the deployment. Using Salad’s public API, Civitai monitors model usage and analyzes the queues, customizing their auto scaling rules to optimize both performance and cost. “Salad not only had the lowest prices in the market for image generation but also offered us incredible scalability. When we needed 200+ GPUs due to a surge in demand, Salad easily met that demand. Plus their technical support has been outstanding” – Justin Maier, Civitai Supporting Millions of unique model combinations on Salad at low cost Civitai’s image generation product supports millions of unique combinations of checkpoints, Low-Rank Adaptations (LoRAs), Variational Autoencoders (VAEs), and Textual Inversions. Users often combine these into a single image generation request. In order to efficiently manage these models on SaladCloud, Civitai combines a robust set of APIs and business logic with a custom container designed to respond dynamically to the image generation demands of their community. At its core, the Civitai image generation product is built around connecting queues with a custom Stable Diffusion container on Salad. This allows the system to gracefully handle surges in image generation requests and millions of unique combinations of models. Each container includes a Python Worker that communicates with Civitai’s Orchestrator. The Worker application is responsible for downloading models, automating image generation with a sequence of calls to a custom image generation pipeline, and uploading resulting images back to Civitai. By building a generic application that is controlled by the Civitai Orchestrator, the overall system automatically responds to the latest trending models and eliminates the need to manually deploy individual models. If an image generation request is received for a combination of models that are already loaded on one or more nodes, the worker will process that request as soon as the GPU is available. If the request is for a combination of models that are not currently loaded into a worker, the job is queued up until the models are downloaded and loaded, then the job is processed. Civitai & Salad – A perfect match for democratizing AI “We chose
Cost-effective Stable Diffusion fine tuning on Salad
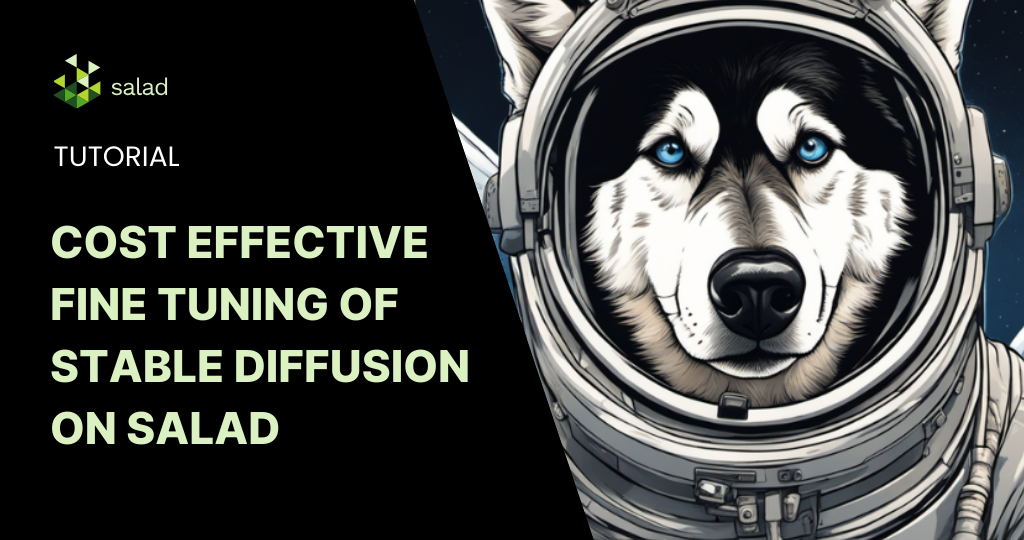
Stable Diffusion XL (SDXL) fine tuning as a service I recently wrote a blog about fine tuning Stable Diffusion XL (SDXL) on interruptible GPUs at low cost, starring my dog Timber. The strong results and exceptional cost performance got me wondering: What would it take to turn that into a fully managed Stable Diffusion training platform? I built one on SaladCloud to find out. The result? Costs as low as $0.00016179 per training step while successfully completing 1000/1000 training jobs. Challenges in developing a Stable Diffusion API There are a number of challenges involved when developing and deploying what’s essentially a Stable Diffusion XL training API on a distributed cloud like Salad. Salad is a distributed cloud with a Million+ individual PCs around the world connected to our network. The GPUs on Salad are latent Nvidia RTX/GTX series. Our goal is for the service to be resilient at any kind of scale. Architecture To handle node interruptions and concurrent training, we built a simple orchestration API, with training compute handled by GPU worker nodes. Additionally, we setup a simple autoscaler using a scheduled Cloudflare Worker. Except for the pool of training nodes, the entire platform uses Cloudflare serverless services. Heavily leveraging serverless technologies for the platform layer greatly reduces operational labor, makes the platform nearly free at rest, and will comfortably scale to handle significantly more load. Given sufficient continuous load, serverless applications do tend to be more expensive than alternatives, so feel free to swap out components as desired. This design doesn’t rely on any provider-specific features, so any SQL database and any Key-Value store would work just as well. API components GPU worker node components Distributing work To get work, worker nodes make a GET request to the API, including their machine id as a query parameter. The API prioritizes handing out that are in the running state, but stalled as measured by heartbeat timeout. It also will never hand a job out to a node where that job has previously failed. Marking a job failed Handling bad nodes If a particular node has failed too many jobs, we want to reallocate it. Our first implementation did not take this into account, and one bad node marked 85% of the benchmark failed, just pulling and failing one job after another. We now run a scheduled Cloudflare Worker every 5 minutes to handle reallocating any nodes with more than the allowed number of failures. Autoscaling the worker pool Our scheduled Cloudflare Worker also handles scaling the worker cluster. It essentially attempts to keep the number of replicas equal to the number of running and pending jobs, with configurable limits. Observing a training run The training script we used from diffusers has a built-in integration with Weights and Biases, a popular ML/AI training dashboard platform. It lets you qualitatively observe the training progress, tracks your training arguments, monitors system usage, and more. Deployment on Salad Deploying on Salad is simple. The worker pattern means we don’t need to enable inbound networking or configure any probes. The only environment configuration needed is a URL for the orchestration API, a key for the orchestration API, and an API key for Weights and Biases (optional). Seeding the benchmark To get a baseline idea of performance, we ran 1000 identical training jobs, each 1400 steps, with text encoder training. We skipped reporting samples to Weights and Balances for this benchmark. We let the auto-scaler run between 0 and 40 nodes each with 2 vCPU, 16GB RAM, and an RTX 4090 GPU. Visualizing a training run Here’s an example training job that got interrupted twice, and was able to resume and complete training on a different node each time. The smaller marks are heartbeat events emitted by the worker every 30s, color coded by machine id. We can see for this run that it sat in the queue for 5.4 hours before a worker picked it up, and ran for 54:00 of billable time, calculated as number of heartbeats * 30s. Plugging that into the Pricing Calculator, we see a cost of $0.324/hour, so a total cost of $0.2916 to train the model and the text encoder for 1400 steps. This comes out to $**0.000208/**step. The amount of time taken, and therefore the cost, varies greatly based on the parameters you use for training. Training the text encoder slows down training. Using prior preservation also slows down training. More steps takes longer. It’s interesting to note that although the run was interrupted multiple times, these interruptions cost less than 4 minutes of clock time, and the run still finished in the median amount of time. Results from the Stable Diffusion XL fine tuning Tips and Observations Future Improvements Conclusions Our exploration into fine-tuning Stable Diffusion XL on interruptible GPUs has demonstrated the feasibility and efficiency of our approach, despite the significant challenges posed by training interruptions, capacity limitations, and cost management. Leveraging Cloudflare’s serverless technologies alongside our custom orchestration and autoscaling solutions, we’ve created a resilient and manageable system capable of handling large-scale operations with notable cost efficiency and operational simplicity. The successes of our deployment, underscored by the seamless completion of 1000/1000 benchmark jobs, highlight the system’s robustness and the potential for further improvements. Future enhancements, such as asynchronous validation and refined node performance assessments, promise to elevate the performance and cost-effectiveness of our service. Given the extensive amount of experimentation required to get good results, a platform like this can be useful for individuals as well as those seeking to build commercial offerings. Once deployed, a person could submit many different combinations of parameters, prompts, and training data, and run many experiments in parallel. Resources Shawn RushefskyShawn Rushefsky is a passionate technologist and systems thinker with deep experience across a number of stacks. As Generative AI Solutions Architect at Salad, Shawn designs resilient and scalable generative ai systems to run on our distributed GPU cloud. He is also the founder of Dreamup.ai, an AI image generation tool that donates 30% of its
Fine tuning Stable Diffusion XL (SDXL) with interruptible GPUs and LoRA for low cost
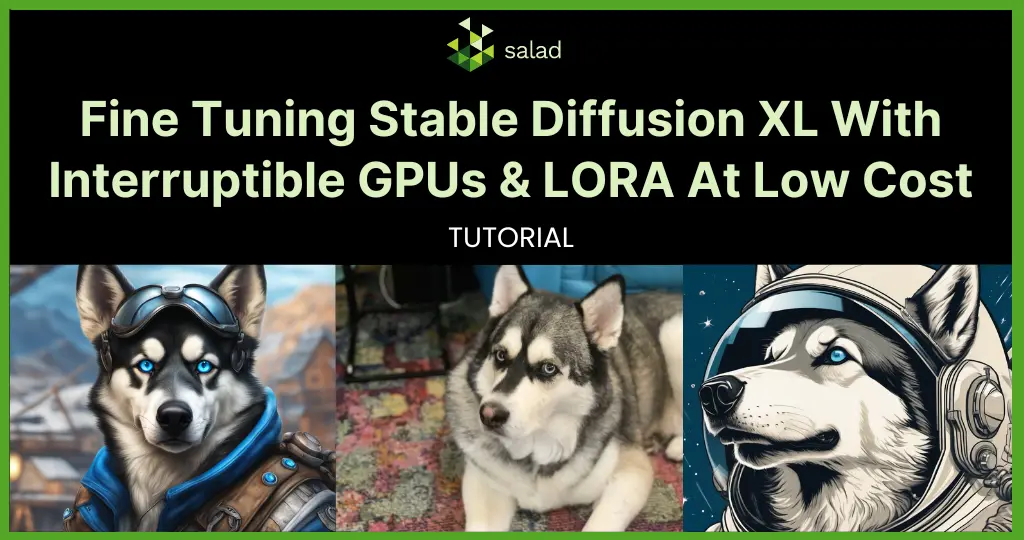
It’s no secret that training image generation models like Stable Diffusion XL (SDXL) doesn’t come cheaply. The original Stable Diffusion model cost $600,000 USD to train using hundreds of enterprise-grade A100 GPUs for more than 100,000 combined hours. Fast forward to today, and techniques like Parameter-Efficient Fine Tuning (PEFT) and Low-Rank Adaptation (LoRA) allow us to fine tune state-of-the-art image generation models like Stable Diffusion XL in minutes on a single consumer GPU. Using spot instances or community clouds like Salad reduces the cost even further. In this tutorial, we fine tune SDXL on a custom image of Timber, my playful Siberian Husky. Benefits of spot instances to fine tune SDXL Spot instances allow cloud providers to sell unused capacity at lower prices, usually in an auction format where users bid on unused capacity. Salad’s community cloud comprises of tens of thousands of idle residential gaming PCs around the world. On AWS, using an Nvidia A10G GPU with the g5.xlarge instance type costs $1.006/hr for on-demand pricing, but as low as $0.5389/hr for “spot” pricing. On Salad, an RTX 4090 (24GB) GPU with 8 vCPUs and 16GB of RAM costs only $0.348/hr. In our tests, we were able to train a LoRA for Stable Diffusion XL in 13 minutes on an RTX 4090, at a cost of just $0.0754. These low costs open the door for increasingly customized and sophisticated AI image generation applications. Challenges of fine tuning Stable Diffusion XL with spot instances There is one major catch, though: both spot instances and community cloud instances have the potential to be interrupted without warning, potentially wasting expensive training time. Additionally, both are subject to supply constraints. If AWS is able to sell all of their GPU instances at on-demand pricing, there will be no spot instances available. Since Salad’s network is residential, and owned by individuals around the world, instances come on and offline throughout the day as people use their PCs. But with a few extra steps, we can take advantage of the huge cost savings of using interruptible GPUs for fine tuning. Solutions to mitigate the impact of interrupted nodes The #1 thing you can do to mitigate the impact of interrupted nodes is to periodically save checkpoints of the training progress to cloud storage, like Cloudflare R2 or AWS S3. This ensures that your training job can pick up where it left off in the event it gets terminated prematurely. This periodic checkpointing functionality is often offered out-of-the-box by frameworks such as 🤗 Accelerate, and simply needs to be enabled via launch arguments. For example, using the Dreambooth LoRA SDXL script with accelerate, as we did, you might end up with arguments like this: This indicates that we want to train for 500 steps, and save checkpoints every 50 steps. This ensures that at most, we lose 49 steps of progress if a node gets interrupted. On an RTX 4090, that amounts to about 73 seconds of lost work. You may want to checkpoint more or less frequently than this, depending on how often your nodes get interrupted, storage costs, and other factors. Once you’ve enabled checkpointing with these launch arguments, you need another process monitoring for the creation of these checkpoints, and automatically syncing them to your preferred cloud storage. We’ve provided an example python script that does this by launching accelerate in one thread, and using another thread to monitor the filesystem with watchdog, and push files to S3-compatible storage using boto3. In our case, we used R2 instead of S3, because R2 does not charge egress fees. Other considerations for SDXL fine tuning The biggest callout here is to automate clean up of your old checkpoints from storage. Our example script saves a checkpoint every 10% progress, each of which is 66MB compressed. Even though the final LoRA we end up with is only 23MB, the total storage used during the process is 683MB. It’s easy to see how storage costs could get out of hand if this was neglected for long enough. Our example script fires a webhook at each checkpoint, and another at completion. We set up a Cloudflare Worker to receive these webhooks and clean up resources as needed. Additionally, while the open source tools are powerful and relatively easy to use, they are still quite complex and the documentation is often very minimal. I relied on youtube videos and reading the code to figure out the various options for the SDXL LoRA training script. However, these open source projects are improving at an increasingly quick pace, as they see wider and wider adoption, so the documentation will likely improve. At the time of writing, the 🤗Diffusers library had merged 47 pull requests from 26 authors, just in the last 7 days. Conclusions Modern training techniques and interruptible hardware combine to offer extremely cost effective fine tuning of Stable Diffusion XL. Open source training frameworks make the process approachable, although documentation could be improved. You can train a model of yourself, your pets, or any other subject in just a few minutes, at a cost of pennies. Training costs have plummeted over the last year, thanks in large part to the rapidly expanding open source AI community. The range of hardware capable of running these training tasks has greatly expanded as well. Many recent consumer GPUs are capable of training an SDXL LoRA model in well under an hour, with the fastest taking just over 10 minutes. Shawn RushefskyShawn Rushefsky is a passionate technologist and systems thinker with deep experience across a number of stacks. As Generative AI Solutions Architect at Salad, Shawn designs resilient and scalable generative ai systems to run on our distributed GPU cloud. He is also the founder of Dreamup.ai, an AI image generation tool that donates 30% of its proceeds to artists.
Stable Diffusion XL (SDXL) Benchmark – 769 Images Per Dollar on Salad
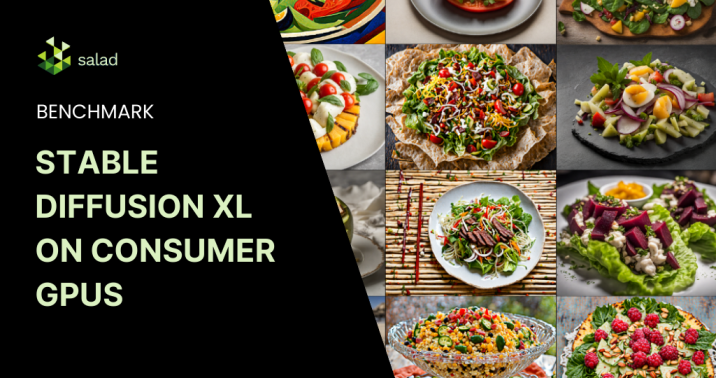
Stable Diffusion XL (SDXL) Benchmark A couple months back, we showed you how to get almost 5000 images per dollar with Stable Diffusion 1.5. Now, with the release of Stable Diffusion XL, we’re fielding a lot of questions regarding the potential of consumer GPUs for serving SDXL inference at scale. The answer from our Stable Diffusion XL (SDXL) Benchmark: a resounding yes. In this benchmark, we generated 60.6k hi-res images with randomized prompts, on 39 nodes equipped with RTX 3090 and RTX 4090 GPUs. We saw an average image generation time of 15.60s, at a per-image cost of $0.0013. At 769 SDXL images per dollar, consumer GPUs on Salad’s distributed cloud are still the best bang for your buck for AI image generation, even when enabling no optimizations on Salad, and all optimizations on AWS. Architecture We used an inference container based on SDNext, along with a custom worker written in Typescript that implemented the job processing pipeline. The worker used HTTP to communicate with both the SDNext container and with our batch framework. Our simple batch processing framework comprises: Discover our open-source code for a deeper dive: Deployment on Salad We set up a container group targeting nodes with 4 vCPUs, 32GB of RAM, and GPUs with 24GB of vram, which includes the RTX 3090, 3090 ti, and 4090. We filled a queue with randomized prompts in the following format: We used ChatGPT to generate roughly 100 options for each variable in the prompt, and queued up jobs with 4 images per prompt. SDXL is composed of two models, a base and a refiner. We generated each image at 1216 x 896 resolution, using the base model for 20 steps, and the refiner model for 15 steps. You can see the exact settings we sent to the SDNext API. Results – 60,600 Images for $79 Over the benchmark period, we generated more than 60k images, uploading more than 90GB of content to our S3 bucket, incurring only $79 in charges from Salad, which is far less expensive than using an A10g on AWS, and orders of magnitude cheaper than fully managed services like the Stability API. We did see slower image generation times on consumer GPUs than on datacenter GPUs, but the cost differences give Salad the edge. While an optimized model on an A100 did provide the best image generation time, it was by far the most expensive per image of all methods evaluated. Grab a fork and see all the salads we made here on our GitHub page. Future Improvements For comparison with AWS, we gave them several advantages that we did not implement in the container we ran on Salad. In particular, torch.compile isn’t practical on Salad, because it adds 40+ minutes to the container’s start time, and Salad’s nodes are ephemeral. However, such a long start time might be an acceptable tradeoff in a datacenter context with dedicated nodes that can be expected to stay up for a very long time, so we did use torch.compile on AWS. Additionally, we used the default fp32 variational autoencoder (vae) in our salad worker, and an fp16 vae in our AWS worker, giving another performance edge to the legacy cloud provider. Unlike re-compiling the model at start time, including an alternate vae is something that would be practical to do on Salad, and is an optimization we would pursue in future projects. Salad Cloud – Still The Best Value for AI/ML Inference at Scale SaladCloud remains the most cost-effective platform for AI/ML inference at scale. The recent benchmarking of Stable Diffusion XL further highlights the competitive edge this distributed cloud platform offers, even as models get larger and more demanding. Shawn RushefskyShawn Rushefsky is a passionate technologist and systems thinker with deep experience across a number of stacks. As Generative AI Solutions Architect at Salad, Shawn designs resilient and scalable generative ai systems to run on our distributed GPU cloud. He is also the founder of Dreamup.ai, an AI image generation tool that donates 30% of its proceeds to artists.
Exploring AI Bias by Turning Faces into Salads – An Experiment
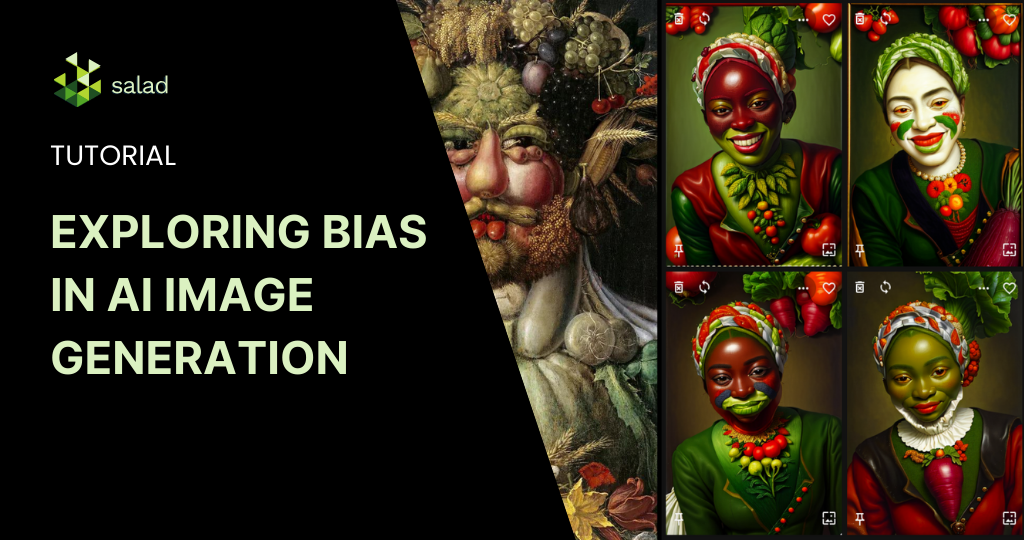
What is AI Bias in Image Generation? Type in ‘An engineer smiling at the camera’ as the prompt into a few AI image generators. What do you see? A collection of men in most cases. In a recent experiment, 298 out of the 300 stable diffusion generated images were of perceived men for the prompt ‘Engineer’. A similar racial/gender bias exists across many AI image generators and models today. AI bias is the phenomenon of artificial intelligence systems producing results that are skewed or unfair towards certain groups or individuals, based on factors such as race, gender, age, or religion. This can occur due to various reasons, such as data bias, algorithm bias or human bias. As the use of AI grows, bias can have serious negative consequences for individuals and society, such as violating human rights, perpetuating social inequalities, and undermining trust in AI systems. While the pervasive bias in AI systems has been spotlighted time and again, especially in the art world, its nuances remain elusive. To explore this, we built our own AI portrait generator from scratch, delving into the subtle ways bias sneaks in and strategizing on crafting prompts to counteract it. Our tool of choice is Dreamup.ai, a completely free AI image generator that shares 30% of its proceeds with artists. We’ll harness the power of the renowned Dreamshaper model. But let’s clear the air first: our pick is based on its stellar image quality and soaring popularity, not because it stands out as more or less biased than its counterparts—it doesn’t. Let’s get started! Creating the AI Portrait Generator For this experiment, we will create a Portrait Generator that transforms any given face into a salad, inspired by the 16th-century Italian painter, Giuseppe Arcimboldo. For this project, we’ll also use ControlNet and Depth Estimation. The process involves taking the 3D structure from an input image and using it, along with a prompt, to produce the final image. This method allows us to create a portrait generator without the need to train a LoRA model from scratch. Step 1: Preparing the Reference Image – Turning a Face into a Salad To start, we’ll use the Dreamshaper model to generate our reference image. Prompt: stunning photograph of a handsome man in a business suit, portrait, city sidewalk background, shallow depth of field Right off the bat, we see a bias issue. The prompt didn’t mention race, but all the generated images are of white men with brown hair, all striking a similar pose with a hand in the left pocket. This lack of diversity stems from the fact that these models are primarily trained on images with English captions, reflecting the biases of English-speaking regions. As our aim is to highlight how biases manifest, let’s adjust our prompt to get a more diverse result. Prompt: stunning photograph of a handsome black man in a business suit, portrait, city sidewalk background, shallow depth of field By specifying race, we get a more diverse image, though the hand-in-pocket pose remains consistent. This suggests that our prompt might be triggering certain cultural or stylistic associations. However, we won’t delve into that now. To ensure authenticity, let’s use a real stock photo as the reference image. Fortune Vieyra in a black business suit, standing by a wall. Source: https://unsplash.com/photos/aAN9ocBHbyo Interestingly, even in this real photo, the hand-in-pocket pose persists. Step 2: Extracting the Depth Map Next, we’ll employ MiDaS Depth Estimation to derive a depth map from our reference image. This depth map will provide us with a 3D perspective of the reference, which is crucial for generating our final artwork. From the depth map, we can discern the structure and layout of the image. However, the color data is absent. This lack of color might pose challenges in the subsequent steps. Depth map derived from our reference image Step 3: Crafting the Perfect Prompt Our first attempt will be a neutral prompt, devoid of any racial or gender specifics. Prompt: Detailed portrait of a person in the ((style of Giuseppe Arcimboldo)), face made of vegetables As expected, the generated images predominantly feature white men, maintaining the composition of the reference. This could be due to the inherent model bias or the historical context of Arcimboldo, a European artist from the 16th century. To achieve our desired outcome, we’ll need to refine our prompt. Prompt: Detailed portrait of a black man in the ((style of Giuseppe Arcimboldo)), face made of vegetables By specifying the race, we get closer to our reference image. However, the overall image is notably darker. This might be due to the encoding process of the text, which can lose intricate details. Prompt: Detailed portrait of an African American man in the ((style of Giuseppe Arcimboldo)), face made of vegetables Switching “black” for “African American” yields a lighter image, but the generated faces are of lighter-skinned individuals. This could reflect a broader spectrum of skin tones or a potential cultural bias. Prompt: Detailed portrait of an african man in the ((style of Giuseppe Arcimboldo)), face made of vegetables Requesting an “african” man gives us darker skin tones, but the backgrounds seem less opulent. This might inadvertently introduce cultural stereotypes. Also, the vegetable face aspect is still missing. Prompt: Detailed portrait of an african aristocratic man in the ((style of Giuseppe Arcimboldo)) Incorporating “aristocratic” brings back the grandeur in the backgrounds. Yet, the vegetable face remains elusive. Prompt: Detailed ((vegetable painting)) of an african aristocratic man in the ((style of Vertumnus by Guiseppe Arcimboldo)) Negative Prompt: (((skin))) Despite multiple attempts, achieving a vegetable-composed face proves challenging. It might be beneficial to use a closer reference image focusing solely on the face for better results. New Reference Image, Depth Map and Prompt Reference image (Photo by Prince Akachi on Unsplash) and New Depth Map With a fresh reference image of a young black woman and an updated depth map, our goal remains: a vegetable-composed face. Prompt: Detailed ((vegetable painting)) of an african aristocratic woman in the ((style
Optimizing AI Image Generation: Streamlining Stable Diffusion with ControlNet in Containerized Environments
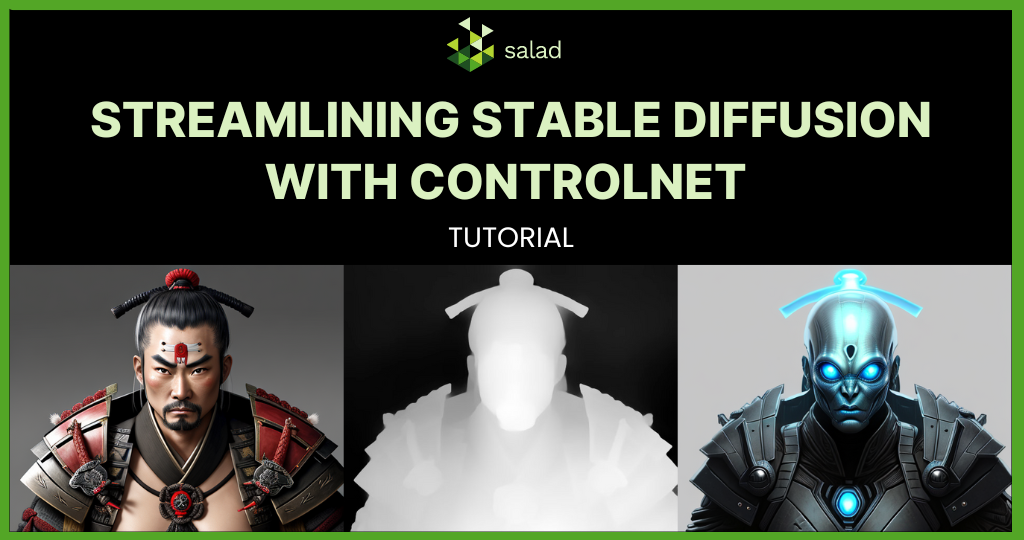
Implementing Stable Diffusion with ControlNet in any containerized environment comes with a plethora of challenges, mainly due to the sizable amount of additional model weights required to be incorporated in the image. In this blog, we discuss these challenges and how they can be mitigated. What is ControlNet for Stable Diffusion? ControlNet is a network structure that empowers users to manage diffusion models by setting extra conditions. It gives users immense control over the images they generate using Stable Diffusion, using a single reference image without noticeably inflating VRAM requirements. ControlNet has revolutionized AI image generation, demonstrating a key advantage of open models like Stable Diffusion against proprietary competitors such as Midjourney. ControlNet for Stable Diffusion: Reference Image (left), Depth-Midas (middle), Output Image (right) Note how the image composition remains the same between the reference image and the final image. This is accomplished by using the depth map as a control image. Images from Dreamup.ai using the Dreamshaper model, MiDas Depth Estimation, and the depth controlnet. Challenges in Implementing ControlNet for Stable Diffusion However, this remarkable feature presents challenges. There are (at the time of this writing) 14 distinct controlnets compatible with stable diffusion, each offering unique control over the output, necessitating a different “control image.” All these models, freely accessible on Huggingface, in separate repositories, amount to roughly 4.3GB each, totaling up to an additional storage need of 60.8GB. This represents a near tenfold increase compared to a minimal Stable Diffusion image. Furthermore, each ControlNet model comes with one or more image preprocessors used to decipher the “control image.” For instance, generating a depth map from an image is a prerequisite for one of the ControlNets. These additional model weights further bloat the total VRAM requirement, exceeding the capacity of commonly used graphics cards like the RTX3060 12GB. The basic approach to implementing ControlNet in a containerized environment. Optimizing ControlNet Implementation in a Containerized Environment on a Distributed Cloud Building a container image for continuous ControlNet stable diffusion inference, if approached without optimization in mind, can rapidly inflate in size. This results in prohibitively long cold-start times as the image is downloaded onto the server running it. This problem is prominent in data centers and becomes even more pronounced in a distributed cloud such as Salad, which depends on residential internet connections of varying speed and reliability. A two-pronged strategy can effectively address this issue. Firstly, isolate ControlNet annotation as a separate service or leverage a pre-built service like this one from Dreamup.ai. This division of labor not only reduces VRAM requirements and model storage in the stable diffusion service but also enhances efficiency when creating numerous output images from a single input image and prompt. Secondly, rather than cloning entire repositories for each model, perform a shallow clone of the model repository without git lfs. Then, use wget to selectively download only the necessary weights, as exemplified by this Dockerfile from the Dreamup.ai Stable Diffusion Service. This tactic alone can save more than 40GB of storage. Stable Diffusion-split-service-controlnet The end result? Two services, both with manageable container image sizes. The ControlNet Preprocessor Service, inclusive of all annotator models, sizes up to 7.5GB and operates seamlessly on an RTX3060 12GB. The Stable Diffusion Service, even with every ControlNet packaged in, comes up to 21.1GB, and also runs smoothly on an RTX3060 12GB. Further reductions can be achieved by tailoring what ControlNets to support. For instance, Dreamup.ai excludes MLSD, Shuffle, and Segmentation ControlNets in their production image, thereby saving about 4GB of storage. Shawn RushefskyShawn Rushefsky is a passionate technologist and systems thinker with deep experience across a number of stacks. As Generative AI Solutions Architect at Salad, Shawn designs resilient and scalable generative ai systems to run on our distributed GPU cloud. He is also the founder of Dreamup.ai, an AI image generation tool that donates 30% of its proceeds to artists.