AI batch transcription benchmark: Speech-to-text at scale
Building upon the inference benchmark of Parakeet TDT 1.1B for YouTube videos on SaladCloud and with our ongoing efforts to enhance the system architecture and implementation for batch jobs, we successfully transcribed over 66,000 hours of YouTube videos using a SaladCloud container group consisting of 100 replicas running for 10 hours. Through this approach, we achieved a cost reduction of 1000-fold while maintaining the same level of accuracy as managed transcription services.
In this deep dive, we will delve into what the system architecture, performance/throughput, time, and cost would look like if we were to transcribe 1 Million hours of YouTube videos. Prior to the test, we created the dataset based on publicly available videos on YouTube. This dataset comprises over 4 Million video URLs sourced from more than 5000 YouTube channels, amounting to approximately 1.6 million hours of content. For detailed methods of collecting and processing data from YouTube on SaladCloud, as well as the reference design and example code, please refer to the guide.
System architecture for AI batch transcription pipeline
The transcription pipeline comprises:
- Job Filler: It filters, generates, and injects jobs based on collected video URLs into the job queue. It also monitors the transcription pipeline process and ensures a consistent supply of tasks by replenishing them regularly.
- Job Queue System: The job queue, like AWS SQS, keeps the video URLs and where to store the generated assets (Cloudflare R2 URLs) for the Salad nodes to retrieve and process.
- YouTube and its CDN: YouTube utilizes a Global Content Delivery Network (CDN) to distribute content efficiently. The CDN edge servers are strategically dispersed across various geographical locations, serving content in close proximity to users and enhancing the speed and performance of applications.
- GPU Resource Pool and Global Distributed Network: Hundreds of Salad nodes equipped with dedicated GPUs are utilized for tasks such as downloading/uploading, pre-processing/post-processing, and transcribing. These nodes assigned to GPU workloads are positioned within a global, high-speed distributed network infrastructure and can effectively align with YouTube’s Global CDN, ensuring optimal system throughput.
- Cloud Storage: Generated assets stored in Cloudflare R2, which is AWS S3-compatible and incurs zero egress fees.
- Job Recording System: Job results, including YouTube video URLs, audio length, processing time, Cloudflare URLs, word count, etc., are stored in NoSQL databases like AWS DynamoDB.
- Job Checker: Validates outcomes using both the metadata files and the job results. The videos that failed to be transcribed can be further analyzed and may be refilled into the pipeline for reprocessing. Then, the final output will be delivered based on some YouTube channels or search topics, potentially including index files containing YouTube video URLs paired with their corresponding transcript URLs on Cloudflare. This facilitates easy access to transcribed content for further analysis and utilization.
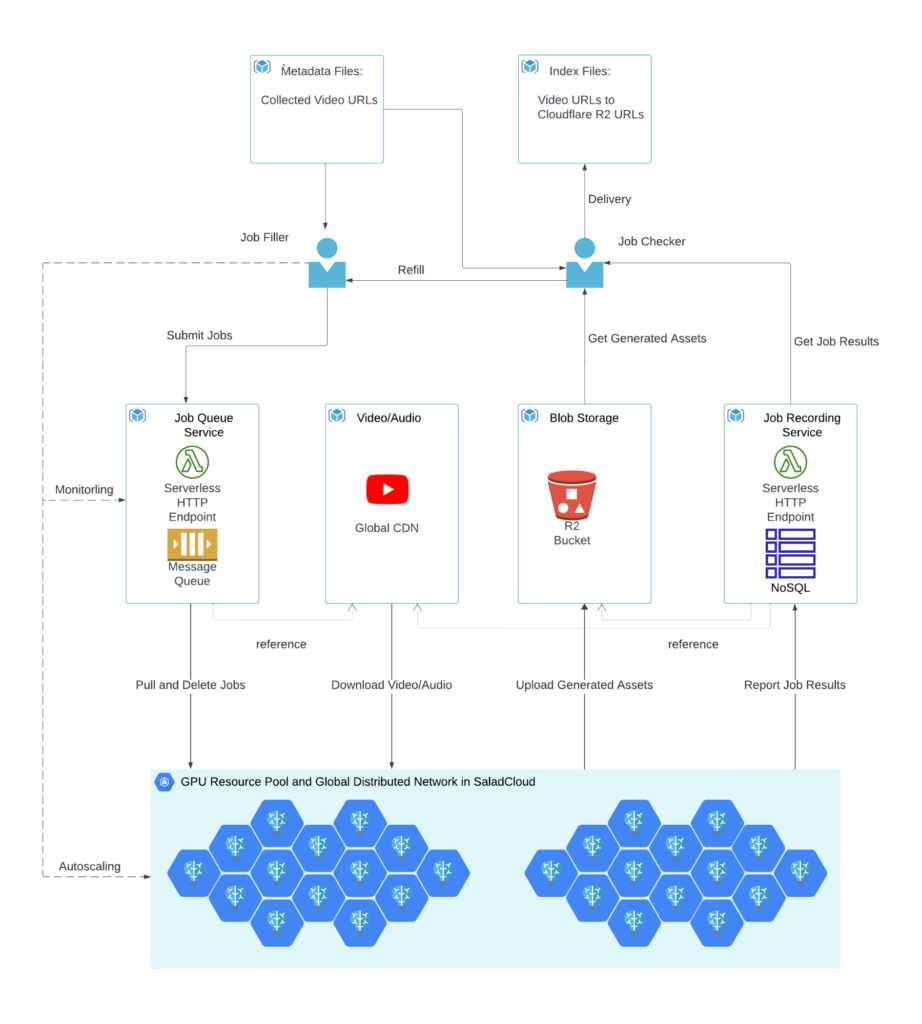
Job Injection Strategy and Job Queue Settings
The provided job filler supports multiple job injection strategies. It can inject millions of hours of video URLs into the job queue instantly and remains idle until the pipeline completes all tasks. However, a potential issue with this approach arises when certain nodes in the pipeline experience downtime and fail to process and remove jobs from the queue. Consequently, these jobs may reappear for other nodes to attempt processing, potentially causing earlier injected jobs to be processed last, which may not be suitable for certain use cases.
For this test, we used a different approach: initially, we injected a large batch of jobs into the pipeline every day and monitored progress. When the queue neared emptiness, we started injecting only a few jobs, with the goal of keeping the number of available jobs in the queue as low as possible for a period of time. This strategy allows us to prioritize completing older jobs before injecting a massive influx of new ones.
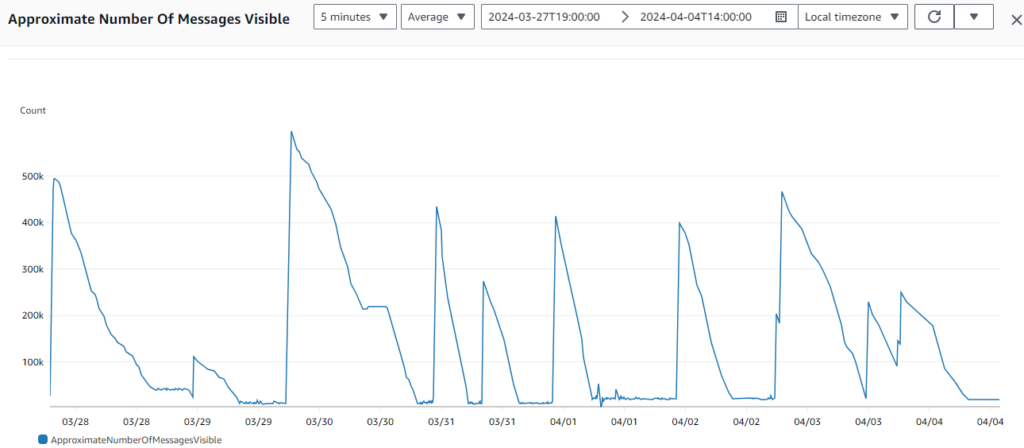
We can also implement autoscaling for time-sensitive tasks. By continually monitoring the job count in the queue, the job filler dynamically adjusts the number of Salad node groups. This adaptive approach ensures that specific quantities of tasks can be completed within a predefined timeframe while also offering the flexibility to manage costs efficiently during periods of reduced demand.
For the job queue system, we set the AWS SQS Visibility Timeout to 1 hour. This allows sufficient time for downloading, chunking, buffering, and processing by most of the nodes in SaladCloud until final results are merged and uploaded to Cloudflare. If a node fails to process and remove polled jobs within the hour, the jobs become available again for other nodes to process.
Additionally, the AWS SQS Retention Period is set to 2 days. Once the message retention quota is reached, messages are automatically deleted. This measure prevents jobs from lingering in the queue for an extended period without being processed for any reason, thereby avoiding wastage of node resources.
Enhanced node implementation
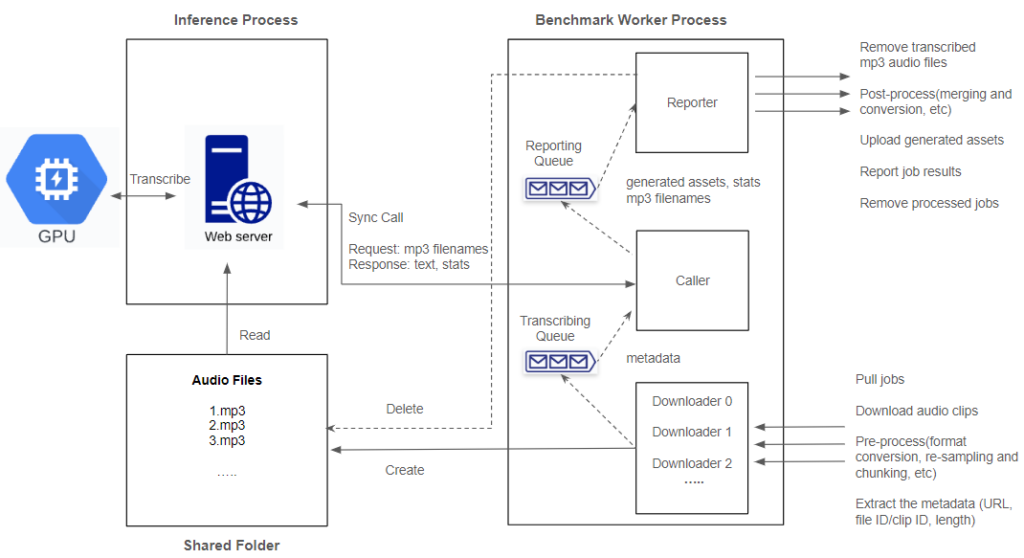
The transcription for audio involves resource-intensive operations on both CPU and GPU, including format conversion, re-sampling, segmentation, transcription, and merging. The more CPU operations involved, the lower the GPU utilization experienced.
Within each node in the GPU resource pool on SaladCloud, we follow best practices by utilizing two processes:
The inference process concentrates on GPU operations and runs on a single thread. It begins by loading the model, warming up the GPU, and then listens on a TCP port by running a Python/FastAPI app on a Unicorn server. Upon receiving a request, it invokes the transcription inference and promptly returns the generated assets.
The benchmark worker process primarily handles various I/O- and CPU-bound tasks, such as downloading/uploading, pre-processing, and post-processing. To maximize performance with better scalability, we adopt multiple threads to concurrently handle various tasks, with two queues created to facilitate information exchange among these threads.
Thread | Description |
Downloader | It reads metadata from the transcribing queue and subsequently sends a synchronous request, including the audio filename, to the inference server. Upon receiving the response, it forwards the generated texts along with statistics, and the transcribed audio filename to the reporting queue. The simplicity of the caller is crucial as it directly influences the inference performance. |
Caller | The reporter, upon reading the reporting queue, deletes the processed audio files from the shared folder and manages post-processing tasks, including merging results and calculating real-time factor and word count. Eventually, it uploads the generated assets to Cloudflare, reports the job results to AWS DynamoDB, and deletes the processed jobs from AWS SQS. |
Reporter | The reporter, upon reading the reporting queue, deletes the processed audio files from the shared folder and manages post-processing tasks, including merging results and calculating real-time factor and word count. Eventually, it uploads the generated assets to Cloudflare, reports the job results to AWS DynamoDB and deletes the processed jobs from AWS SQS. |
By running two processes to segregate GPU-bound tasks from I/O and CPU-bound tasks and fetching and preparing the next audio clips concurrently and in advance while the current one is still being transcribed, we can eliminate any waiting period. After one audio clip is completed, the next is immediately ready for transcription. This approach not only reduces the overall processing time for batch jobs but also leads to even more significant cost savings.
1 million hours of YouTube video batch transcription tests on SaladCloud
We established a container group with 100 replicas, each equipped with 2vCPU, 12 GB RAM, and a GPU with 8GB or more VRAM on SaladCloud. This group remained operational for 180 hours (equivalent to 7.5 days), starting from 20:00 on the preceding Wednesday and concluding at 14:00 on the subsequent Thursday afternoon. During this period, we temporarily halted the pipeline for approximately 6 hours to implement changes in node implementation for testing various algorithms. Additionally, videos of specific and large lengths were injected at the final stage to evaluate the pipeline’s performance and throughput under specific conditions.
Based on the AWS DynamoDB metrics, particularly the writes per second, which function as a monitoring tool for the number of transcribed videos per second for the transcription pipeline, the curve exhibited significant fluctuations due to the random distribution of video lengths within the dataset. However, stability was achieved after injecting specific and large lengths of videos on April 4th.

Breakdown of batch transcription by date on SaladCloud
During the 180-hour period, the pipeline, consisting of 100 replicas of Salad nodes, successfully transcribed 1,001,812 hours of YouTube videos. Here is the breakdown of numbers:
Date | Total Hours Transcribed | Total Video Number | Average Video Length (second) | Average Real-Time Factor | Hours of Data |
---|---|---|---|---|---|
2024-03-27 | 19,692 | 101,939 | 695 | 86 | 4 |
2024-03-28 | 133,689 | 402,083 | 1197 | 85 | 24 |
2024-03-29 | 136,856 | 372,204 | 1324 | 85 | 24 |
2024-03-30 | 100,507 | 533,037 | 679 | 80 | 18 |
2024-03-31 | 128,795 | 491,854 | 943 | 83 | 24 |
2024-04-01 | 119,394 | 506,752 | 848 | 80 | 24 |
2024-04-02 | 130,219 | 445,291 | 1053 | 82 | 24 |
2024-04-03 | 141,670 | 311,292 | 1638 | 88 | 24 |
2024-04-04 | 90,990 | 89,572 | 3657 | 89 | 14 |
1,001,812 | 3,254,024 | 1108 | 83 | 180 |
As expected, the correlation between the pipeline throughput and performance with the injected video lengths is evident: longer videos generally lead to better performance. Minor variances in the average real-time factor, calculated as the audio length divided by the processing time and serving as an efficient measure of transcription performance, are observed around the video length of 1000 seconds.
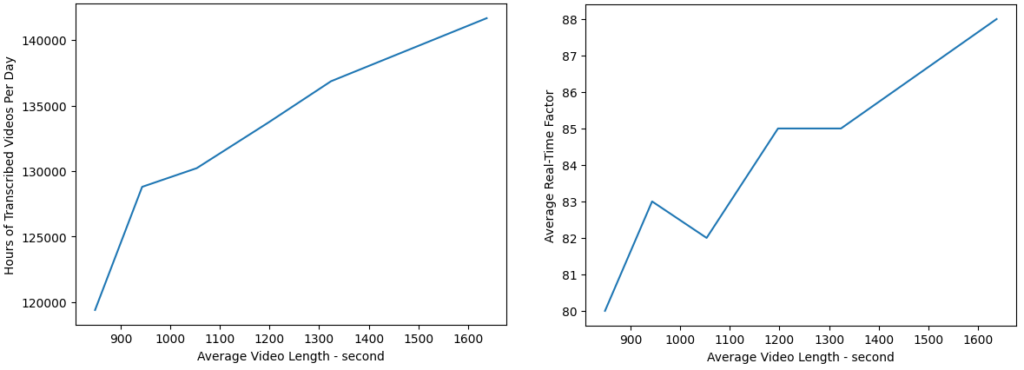
The throughput may not consistently increase with longer video lengths. For instance, if a 10-hour video is downloaded and chunked into 60 10-minute segments by a node, and the node encounters an issue while processing the final segments, the job for this video will reappear in the job queue. This scenario can potentially lead to resource wastage as other nodes attempt to download and process the video again.
Benchmark results comparing audio, video (scaled), and batch video transcription on SaladCloud
Let’s compare the results of the three benchmark tests conducted on Parakeet TDT 1.1B for audio and video:
Parakeet Audio | Parakeet YouTube Video (66k+ hrs scaled to 1M by a factor) | Parakeet YouTube Video (1 Million+ hrs) | |
---|---|---|---|
Duration | 10 hours | 10 hours | 180 hours |
Datasets | English CommonVoice and Spoken Wikipedia Corpus English | Collected YouTube videos from 5000+ channels, more than 1.6 million hours, and 4 million video URLs. | Around $100 100 Replicas (2vCPU,12GB RAM, GPU with 8 GB or more VRAM) |
Average Length (s) | 12 | 3515 | 1108 |
Cost on SaladCloud (GPU Resource Pool and Global Distribution Network) | Around $100 100 Replicas (2vCPU,12GB RAM, GPU with 8 GB or more VRAM) | Around $1800 100 Replicas (2vCPU,12GB RAM, GPU with 8 GB or more VRAM) | Around $1800 100 Replicas (2vCPU,12GB RAM,GPU with 8 GB or more VRAM) |
Cost on AWS and Cloudflare (Job Queue/Recording System and Cloud Storage ) | Around $20 | Around $2 | Around $25 |
Node Implementation | 3 downloader threads; Segmentation of long audio; Merging texts. | Download audio from YouTube videos; 3 downloader threads; Segmentation of long audio; Format conversion from Mp4a to MP3; Merging texts. | Poll and delete jobs from AWS SQS; Download audio YouTube videos; 3 downloader threads; Adaptive Buffer Size for waiting jobs; Segmentation of long audio; Format conversion from Mp4a to MP3; Merging texts; Real-time performance check; Upload generated assets to Cloudflare |
Numbers of Transcribed Audio/Video | 5,209,130 | 68,393 | 3,254,024 |
Total Length (s) | 62,299,198 (17,305 hours) | 240,427,792 (66,786 hours ) | 3,606,523,000 (1,001,812 hours ) |
Most Cost-Effective GPU Type for transcribing long audio | RTX 3070 Ti 794 hours per dollar | RTX 3070 Ti 794 hours per dollar | RTX 3070 Ti 794 hours per dollar |
System Throughput | Downloading and Transcribing 1731 seconds of audio per second. | Downloading and Transcribing 6679 seconds of video per second. | Downloading and Transcribing 5566 seconds of video per second. |
It is worth noting that our previous Parakeet TDT 1.1B benchmark calculated a 'predicted cost' of $1260 to transcribe 1 Million hrs. In that case, we transcribed only 66,786 hrs of video and scaled the cost/hour based on the most cost-effective GPU type to calculate the price for 1 Million hours. In this benchmark, we calculate the 'real cost' to be $1800 by actually transcribing 1 Million hours of audio using a combination of different GPU types.
As we utilize the identical ASR model, Parakeet TDT 1.1B, for transcribing segmented audio files, there isn’t a significant variance in the individual node’s transcribing performance and cost-effectiveness.
However, there are notable disparities in system performance and throughput, largely attributable to input audio/video length. The efficiency of network transmission is notably enhanced when downloading large files compared to numerous small files. Similarly, GPU utilization sees improvements when handling substantial data transfers between the CPU and GPU memory and reducing the frequency of launching GPU kernels.
The quality of the dataset also matters. In the case of the 10-hour YouTube test, all videos were carefully selected, ensuring they were valid for processing. However, during the 180-hour YouTube test, despite applying filtering algorithms to the comprehensive dataset and manual check, some video URLs were unreachable, and some videos failed to download, chunk, or convert properly. This resulted in wasted time and resources, particularly when videos were already downloaded but failed to be processed further.
Optimization of Cost, Performance, and Throughput
There are various methods to further optimize the results, aiming for reduced costs, improved performance or higher throughput, and different approaches may yield distinct outcomes.
Choosing more cost-effective GPU types in the resource pool will result in additional cost savings. If performance is the priority, selecting higher-performing GPU types is advisable while still remaining significantly less expensive than managed transcription services. Furthermore, by easily increasing the replica number, we can quickly enhance the system throughput, allowing for the efficient handling of time-sensitive tasks.
We can further optimize the node implementation. You may define a resource group on SaladCloud that can encompass various GPU types, and the Salad nodes are distributed across the global Internet, each with varying bandwidth and latency to specific endpoints. Moreover, the performance of each node and the number of running replicas within a group may fluctuate over time due to their shared nature.
Best practices for optimizing batch transcription on SaladCloud
To optimize node performance and system throughput for your applications, consider the following best practices:
Define Initialization Time Threshold: Establish a threshold for initialization time, encompassing tasks such as model loading and warm-up. If a node’s initialization time exceeds this threshold, such as 300 seconds, the code should exit. SaladCloud will allocate a new node in response, ensuring that nodes are adequately prepared to execute your application.
Monitor Real-Time Performance: Continuously monitor application performance within a specific time window. If performance metrics, such as the real-time factor, fall below a predefined threshold, the code should exit, prompting reallocation. This practice ensures that nodes remain in an optimal state for application execution.
Adopt Adaptive Algorithms: Recognize that nodes may vary in GPU types and network performance. High-performance nodes can handle longer chunks and batch inference with a large buffer size for waiting jobs, whereas low-performance nodes are better suited for processing shorter chunks with a smaller buffer size. By employing adaptive algorithms, resource utilization can be optimized while preventing low-performance nodes from impeding overall progress.
Performance Comparison across Different Clouds
By harnessing the high-speed ASR model – Parakeet TDT 1.1B and leveraging thousands of Consumer GPUs on SaladCloud, along with the provided the guide and example code, you can swiftly build and operate your own transcription infrastructure. This enables the delivery of transcription services at a massive scale and at an extremely low cost, while maintaining the same level of accuracy as managed transcription services.
While adopting the most cost-effective GPU type for the inference of Parakeet TDT 1.1B on SaladCloud, $1 dollar can transcribe 47,638 minutes of audio (nearly 800 hours), showcasing over 1000-fold cost reduction compared to other public cloud providers.

SaladCloud: The Most Affordable GPU Cloud for Batch Jobs
Consumer GPUs are considerably more cost-effective than Data Center GPUs. While they may not be the ideal choice for extensive and large model training tasks, they are powerful enough for the inference of most AI models. With the right design and implementation, we can build a high-throughput, reliable and cost-effective system on top of them, offering massive inference services.
If your requirement involves hundreds of GPUs for large-scale inference or handling batch jobs on global networks, or you need tens of different GPU types within minutes for testing or research purposes, SaladCloud is the ideal platform.