Text-to-Speech (TTS) API Alternative: Self-Managed OpenVoice vs MetaVoice Comparison
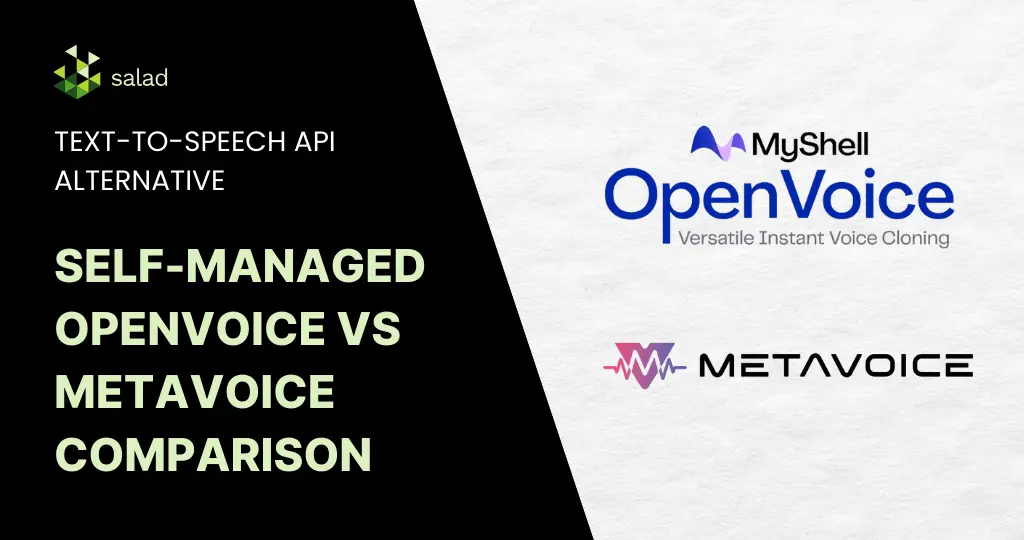
A cost-effective alternative to Text-to-speech APIs In the realm of text-to-speech (TTS) technology, two open-source models have recently garnered everyone’s attention: OpenVoice and MetaVoice. Each model has unique capabilities in voice synthesis, but both were recently open sourced. We conducted benchmarks for both models on SaladCloud showing a world of efficiency and cost-effectiveness, highlighting the platform’s ability to democratize advanced voice synthesis technologies. The benchmarks focused on self-managed OpenVoice and MetaVoice as a far cheaper alternative to popular text to speech APIs. In this article, we will delve deeper into each of these models, exploring their distinctive features, capabilities, price, speed, quality and how they can be used in real-world applications. Our goal is to provide a comprehensive understanding of these technologies, enabling you to make informed decisions about which model best suits your voice synthesis requirements. If you are serving TTS inference at scale, utilizing a self-managed, open-source model framework on a distributed cloud like Salad is 50-90% cheaper compared to APIs. Efficiency and affordability on Salad’s distributed cloud Recently, we benchmarked OpenVoice and MetaVoice on SaladCloud’s global network of distributed GPUS. Tapping into thousands of latent consumer GPUs, Salad’s GPU prices start from $0.02/hour. With more than 1 Million PCs on the network, Salad’s distributed infrastructure provides the computational power needed to process large datasets swiftly, while its cost-efficient pricing model ensures that businesses can leverage these advanced technologies without breaking the bank. Running OpenVoice on Salad comes out to be 300 times cheaper than Azure Text to Speech service. Similarly, MetaVoice on Salad is 11X cheaper than AWS Polly Long Form. A common thread: Open Source Text-to-Speech innovation OpenVoice TTS, OpenVoice Cloning, and MetaVoice share a foundational principle: they are all open-source text-to-speech models. These models are not only free to use but also offer transparency in their development processes. Users can inspect the source code, contribute to improvements, and customize the models to fit their specific needs. With the source code, developers and researchers can customize and enhance these models to suit their specific needs, driving innovation in the TTS domain. A closer look at each model: OpenVoice and MetaVoice OpenVoice is an open-source, instant voice cloning technology that enables the creation of realistic and customizable speech from just a short audio clip of a reference speaker. Developed by MyShell.ai, OpenVoice stands out for its ability to replicate the voice’s tone color while offering extensive control over various speech attributes such as emotion and rhythm. OpenVoice voice replication process involvesseveral key steps that can be used both together or separately: OpenVoice Base TTS OpenVoice’s base Text-to-Speech (TTS) engine is a cornerstone of its framework, efficiently transforming written text into spoken words. This component is particularly valuable in scenarios where the primary goal is text-to-speech conversion without the need for specific voice toning or cloning. The ease with which this part of the model can be isolated and utilized independently makes it a versatile tool, ideal for applications that demand straightforward speech synthesis. OpenVoice Benchmark: 6 Million+ words per $ on Salad OpenVoice Cloning Building upon the base TTS engine, this feature adds a layer of sophistication by enabling the replication of a reference speaker’s unique vocal characteristics. This includes the extraction and embodiment of tone color, allowing for the creation of speech that not only sounds natural but also carries the emotional and rhythmic nuances of the original speaker. OpenVoice’s cloning capabilities extend to zero-shot cross-lingual voice cloning, a remarkable feature that allows for the generation of speech in languages not present in the training dataset. This opens up a world of possibilities for multilingual applications and global reach. MetaVoice-1B MetaVoice-1B is a robust 1.2 billion parameter base model, trained on an extensive dataset of 100,000 hours of speech. Its design is focused on achieving natural-sounding speech with an emphasis on emotional rhythm and tone in English. A standout feature of MetaVoice 1B is its zero-shot cloning capability for American and British voices, requiring just 30 seconds of reference audio for effective replication. The model also supports cross-lingual voice cloning with fine-tuning, showing promising results with as little as one minute of training data for Indian speakers. MetaVoice-1B is engineered to capture the nuances of emotional speech, ensuring that the synthesized output resonates with listeners on a deeper level. MetaVoice Benchmark: 23,300 words per $ on Salad Benchmark results: Price comparison of voice synthesis models on SaladCloud The following table presents the results of our benchmark tests, where we ran the models OpenVoice TTS, OpenVoice Cloning, and MetaVoice on SaladCloud GPUs. For consistency, we used the text from Isaac Asimov’s book “Robots and Empire”, available on Internet Archive: Digital Library of Free & Borrowable Books, Movies, Music & Wayback Machine , comprising approximately 150,000 words, and processed it through all compatible Salad GPUs. Model Name Most Cost-EfficientGPU Words per Dollar Second Most CostEfficient GPU Words per Dollar OpenVoice TTS RTX 2070 6.6 Million GTX 1650 6.1 million OpenVoice Cloning GTX 1650 4.7 Million RTX 2070 4.02 million MetaVoice RTX 3080 23,300 RTX 3080 Ti 15,400 Table: Comparison of OpenVoice Text-to-Speech, OpenVoice Cloning and MetaVoice The benchmark results clearly indicate that OpenVoice, both in its TTS and Cloning variants, is significantly more cost-effective compared to MetaVoice. The OpenVoice TTS model, when run on an RTX 2070 GPU, achieves an impressive 6.6 Million words per dollar, making it the most efficient option among the tested models. The price of using RTX2070 on SaladCloud is $0.06/hour which together with vCPU and RAM we used got us to a total of $0.072/hour. OpenVoice Cloning also demonstrates strong cost efficiency, particularly when using the GTX 1650, which processes 4.7 Million words per dollar. This is a notable advantage for applications requiring less robotic voice. In contrast, MetaVoice’s performance on the RTX 3080 and RTX 3080 Ti GPUs yields significantly fewer words per dollar, indicating a higher cost for processing speech. However, don’t rush to dismiss MetaVoice just yet; upcoming comparisons may offer a different perspective that could sway your opinion.
Bark Benchmark: Reading 144K Recipes with Text-to-Speech on SaladCloud
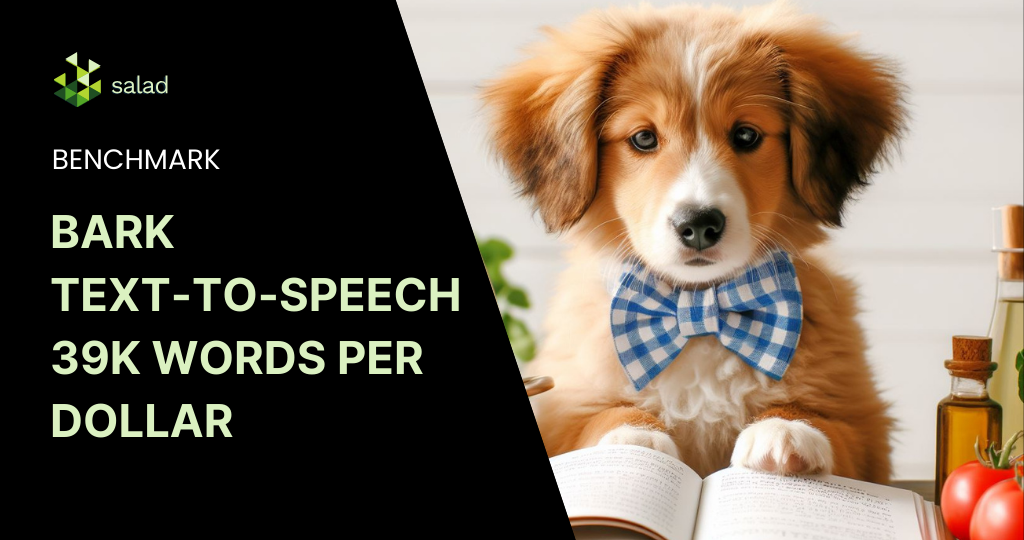
Speech Synthesis with suno-ai/bark When you think of speech synthesis, you might think of a very robotic sounding voice, like this one from 1979. Maybe you think of more modern voice assistants, like Siri or the Google Assistant. While these are certainly improvements over what we had in the 1970s, they still wouldn’t be mistaken for recordings of actual humans. Enter Bark text-to-speech, a generative AI model like Stable Diffusion or ChatGPT developer by Suno AI. Like these other generative models, Bark takes a text prompt, and creates something new. However, it doesn’t produce images, or more text. From their github page: “Bark can generate highly realistic, multilingual speech as well as other audio – including music, background noise and simple sound effects. The model can also produce nonverbal communications like laughing, sighing and crying.” This is a fundamental departure from previous generations of speech synthesis. Bark does not try to break down text into phonemes for recreation by a recorded voice. Rather, it “predicts” what an audio recording might be like, based on the text it’s given. The result is much more natural sounding speech and other conversational sounds. Bark is also an important generative AI model because it is freely available for commercial use, and can run on very modest hardware, including consumer GPUs with minimal vRAM. We set out to benchmark Bark across a range of consumer hardware configurations, using Salad’s GPU Cloud. Benchmarking Bark text-to-speech model on Consumer GPUs You know we like to keep things food related here at Salad, so we selected this Food.com Recipe Dataset from Kaggle, a collection of a couple hundred thousand recipes, along with reviews of those recipes. We’re going to have Bark read these recipes out for us. If you’d like to follow along, we’ll be working with Python 3.10 throughout this project. Unlike some of our other benchmarks, our goal here is not to demonstrate that Salad is the most cost-effective platform for AI inference. Rather, we want to leverage some unique capabilities of Salad’s distributed cloud to evaluate Bark’s performance across a wide range of consumer GPUs. And, if I’m being totally honest, I just thought this would be a fun project. You can skip straight to the outputs if that’s what you’re here for. Architecture We’ll be using our standard batch processing framework for this, the same we’ve used for many other benchmarks, including Whisper Large and SDXL. Data Preparation First, we need to download our dataset. Kaggle is free, but does require an account. Once you have an account, you’ll need to grab your API token from your account settings. Clicking the “Create New Token” button will initiate a download of a file called kaggle.json. Place the file in your home directory at ~/.kaggle/kaggle.json. This will allow you to make authenticated requests with the Kaggle CLI. Now we have a folder called food-com-recipes-and-user-interactions that contains the following files: Our first step is to load up our recipes and interactions in a pandas DataFrame. This step may take several minutes. Let’s take a peek and see what we’re working with. Ok, so we have 231,637 recipes, with fields like “id”, “name”, “description”, and “steps”. There’s some other fields as well, but we won’t be using them for this project. Let’s check out our review data. In our review data, we have 1,132,367 reviews, each of which has a “recipe_id” and a “rating”. Let’s see our top recipes by average review: Interestingly, we see a lot of recipes with an average rating of 0.0. Maybe we should filter this down to only recipes with “good” reviews, over 4.5. Ok, now we’ve got 144,177 recipes that have received an average rating of at least 4.5. Now we can merge this table into the recipe table, and get a collection of recipe data, but only for recipes with a rating of at least 4.5. One thing to note here is that although steps looks like a list of strings, it is in fact just a string. Since our goal is to write a “script” for Bark to read, we’re going to want these strings parsed into lists. We’re going to use the ast module to safely evaluate these strings into python lists. Ok, now we need to turn this data into a “script”: something that will sound a little more natural when Bark reads it. I’ll admit, I was tempted to use a Large Language Model (LLM) like Llama 2 for this, and the results would have likely been better, and more natural sounding. However, for the sake of expediency, I’m just going to use a simple python function to stitch each row into a script. Let’s test it on our first row. This will be good enough for this project. We can see there’s some typos in the original data, and it’ll be interesting to see how Bark handles those. However, we have a new problem now, which is that Bark works best with about 13 seconds of spoken text. Our script is quite a bit longer than that, so we’re going to have to chop it up into smaller chunks. According to a quick google search, the average speaking rate is 2.5 words per second, which would translate to a maximum of 32.5 words that Bark will happily do in one clip. Let’s round that down to 30, just to be safe. However, we don’t just want to split the script every 30 words. Ideally, we would only include whole sentences for each segment, so that Bark can do a better job of tone and cadence. There are Natural Language Processing (NLP) techniques to do this with greater accuracy, but again, for expediency, we’re going to do this the simple way. Let see how that works: Ok, that’s pretty good. Let’s move forward with this solution. Bark includes a large number of voice presets, but since our data is all English, we’re going to use just the English language voices. There’s 10 of those, numbered 0-9.