How to Refactor Public Cloud to Reduce Cloud Cost
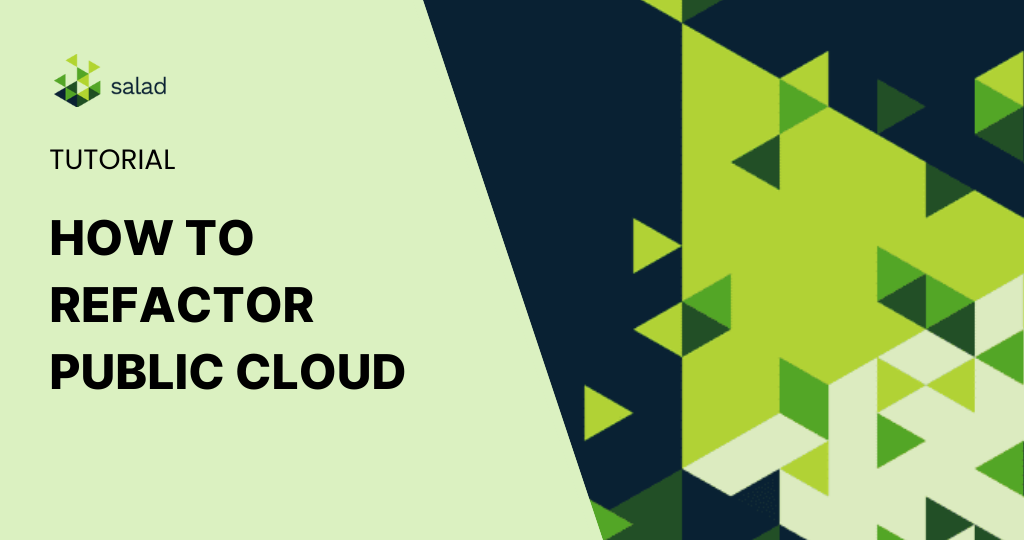
Cloud cost is ballooning for everyone. The global cloud market is expected to generate at least $206B in revenue this year, even as customers overwhelmingly fear overprovisioning and estimate that up to 32% of cloud spend goes to waste. It’s only natural to assume that rational actors will embrace new technologies and providers to lighten the load. Thanks to the broad adoption of containerization, we’re headed for a fragmentation of the landscape, wherein more and more customers may turn to alternative infrastructure. Containers Made Multicloud Possible Nowadays, it’s rare to find a company without a hybrid or multicloud configuration, but that wasn’t always the case. Vendor lock-in used to be a fact of life. Enterprises anxious to modernize during the heyday of Web 2.0 didn’t have the time to compare cloud solutions once they were up and running, and neither did the up-and-comers nipping at their heels. For enterprises and innovators alike, it was oftentimes easier to tack on a service from a current vendor than shop around for the most cost-effective solution. Containerization changed all that. The portability and immutability of the format made it possible to package software with its dependencies and execute as intended wherever it was sent. As web applications decoupled, software developers began to take a renewed interest in optimizing cloud spending by aligning their resource strategies to discrete use cases. The benefits of immutability and portability translate to zero switching cost. Now nearly every cloud customer on the planet relies on multicloud infrastructure powered by containerization technologies and virtual machines. By making better, more needs-based choices, cloud customers came to acknowledge a fundamental flaw in the value proposition of public cloud: complexity inevitably leads to overprovisioning and high cloud costs. Serverless Wasn’t Painless In the last half decade, centralized cloud providers have tried to adapt to reality. The Big Three released a spate of so-called “serverless” container orchestration services that ostensibly sought to address a growing appetite for simplicity. Billed universally as out-of-the-box solutions, these products promised to streamline deployment by eliminating the operational burden of server management and allowing customers to focus solely on app development. I assume we’re all pretty familiar with how marketing works. Leaving aside, for the moment, that “serverless” is a horribly imprecise term of art, the first generation of fully managed orchestration services didn’t do enough to distinguish themselves as standalone solutions. Added perks like on-demand elastic bursting, event-driven autoscaling, and pay-as-you-go pricing certainly sweetened the deal, but folks lost in the wildlands of “everything-as-a-service” couldn’t readily grok the value proposition. If the provider continued to manage the underlying infrastructure, wasn’t serverless just a nuanced version of the conventional cloud deployment workflow? That’s not to say those products had no merit. Fully managed orchestration is a valuable service for specialized and discrete use cases, and reclaimed development time was a sufficient incentive for many notable enterprises to embrace it. But those initial offerings didn’t provide the cost optimization they were after. Fully managed orchestration failed to curry early favor because the first products on the market were too much like everything else. They came laden with fluctuating and extravagant cloud costs, unforeseen operational overhead, and a tendency toward vendor lock-in. Worst of all, they didn’t actually alleviate pain points. They just redistributed them! Frustration-as-a-Service No category of cloud products has fallen short of its promise quite like fully managed serverless. Instead of streamlining deployment and unburdening developers, most of those early solutions inadvertently replaced the onus of server management with a multitude of additional tasks. As Corey Quinn of Last Week in AWS explains: The bulk of your time building serverless applications will not be spent writing the application logic… Instead you’ll spend most of your time figuring out how to mate these functions with other services from that cloud provider. In practice, functions-as-a-service (FaaS) products like AWS Lambda require such declarative execution patterns that they transmute hours better spent on business logic into a full-time business of managing functions to get the job done as intended. (You could argue that Lambda does help to reduce a codebase to its essential business logic, but that’s like saying the monkey’s paw really did grant wishes.) Can you blame anyone for dismissing fully managed orchestration from the start? Detractors looked at the gooey, “serverless” moniker as a gateway drug to vendor lock-in, while more charitable critics begrudgingly admitted that it could be a stopgap solution for cases where your go-live was yesterday, but it wasn’t something you’d choose to rely on in the long haul. I’m inclined to agree—at least as the assessment pertains to those early implementations. Engaging with centralized serverless invariably forced you to sacrifice autonomy, limit versatility, and incur unnecessary expense. Put another way, they suffered the classic ills of public cloud. The CaaS Alternative To my mind, every industry trend suggests a prevailing sense of cloud fatigue: Budgetary spillover has undermined the whole argument for leaving bare metal in the first place! Now that containers have broken the floodgates, customers are well provisioned to divert resources to novel infrastructure that’s more optimized for containerized deployment. And there’s nothing more frightening to tier-one vendors than simple, affordable alternatives. For evidence, you’d need only consider the newest fully managed products from the Big Three. Long gone are the daisy-chained function outputs and thorny, plug-and-play backends. Newer containers-as-a-service (CaaS) products like AWS Fargate and Azure Container Instances have reduced the feature set to exactly what’s on the box—namely infrastructure, orchestration, and event-driven scaling. If that sounds like “Docker images on demand,” that’s because it is. It could even be the future. Companies don’t want to spend inordinate amounts monitoring systems that cost peanuts to run. Fully managed CaaS orchestration is especially useful for discrete use cases that don’t require access to the underlying nodes, such as long-running data processing batch jobs or 3-D accelerated rendering queues, but it’s equally suited to autoscaling decoupled microservices. In a recent survey by Datadog, a significant share of cloud customers signaled interest in migrating Kubernetes clusters to fully managed serverless. That’s great news for the paradigm as a whole, but
Stable Diffusion v1.4 Inference Benchmark – GPUs & Clouds Compared
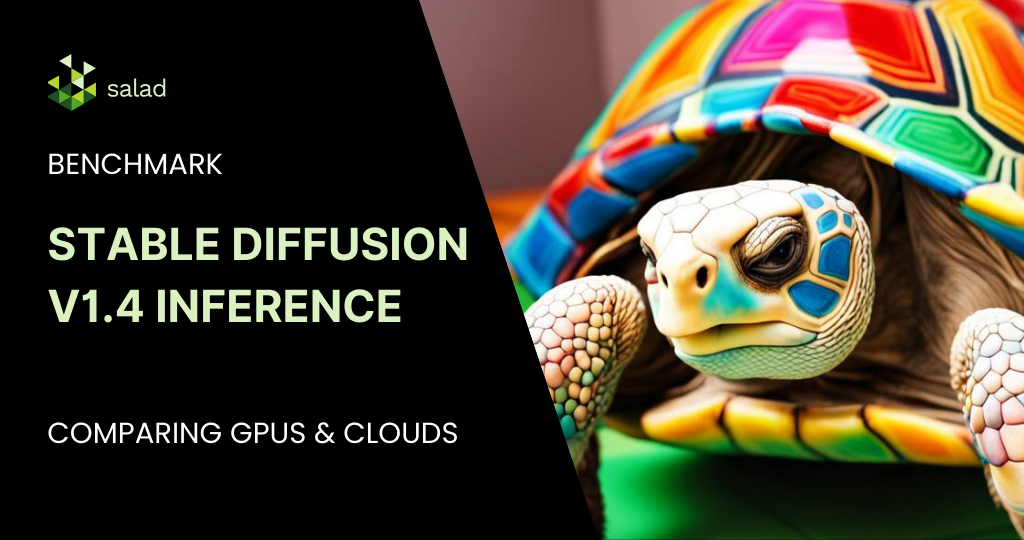
Stable Diffusion v1.4 GPU Benchmark – Inference Stable Diffusion v1.4 is an impressive text-to-image diffusion model developed by stability.ai. By utilizing the principles of diffusion processes, Stable Diffusion v1.4 produces visually appealing and coherent images that accurately depict the given input text. Its stable and reliable performance makes it a valuable asset for applications such as visual storytelling, content creation, and artistic expression. In this benchmark, we evaluate the inference performance of Stable Diffusion 1.4 on different compute clouds and GPUs. Our goal is to answer a few key questions that developers ask when deploying a stable diffusion model to production: Benchmark Parameters For the benchmark, we compared consumer-grade, mid-range GPUs on two community clouds – SaladCloud and Runpod with higher-end GPUs on three big-box cloud providers. To deploy on SaladCloud, we used the 1-click deployment for Stable Diffusion (SD) v1.4 on the Salad Portal via pre-built recipes. Cloud providers considered: Google Cloud Platform (GCP), Amazon Web Services (AWS), Microsoft Azure Cloud, RunPod and SaladCloud. GPUs considered RTX 3060, RTX 3090, A100, V100, T4, RTX A5000 Link to model: https://huggingface.co/CompVis/stable-diffusion-v1-4 Prompt: ‘a bowl of salad in front of a computer’ The benchmark analysis uses a text prompt as input. Outputs were images in the 512×512 resolution with 50 inference steps as recommended in this HuggingFace blog. Image: A bowl of Salad in front of a computer – generated from the benchmark For the comparison, we focused on two main criteria: Images Per Dollar (Img/$) Training stable diffusion definitely needs high-end GPUs with high vRAM. But for inference, the more relevant metric is Images Per Dollar. There have been multiple instances of rapid user growth for a text-to-image platform either causing skyrocketing cloud bills or a mad scramble for GPUs. A high number of images generated per dollar means cloud costs are lower and generative AI companies can grow at scale in a profitable manner. Seconds Per Image (sec/img) The user base for SD-based image generation tools are vastly different when it comes to image generation time. In some cases, end-users expect images in under 5 seconds (Dall-e, Canva, Picfinder, etc). In others like Secta.ai, users expect results in a few minutes to hours. The image generation times can also vary for different pricing tiers. Free tier users can expect to wait a couple more seconds compared to users paying the highest price for access. Stable Diffusion GPU Benchmark – Results Image: Stable Diffusion benchmark results showing a comparison of images per dollar for different GPUs and clouds The benchmark results show the consumer-grade GPUs outperforming the high-end GPUs, giving more images per dollar with a comparable image generation time. For generative AI companies serving inference at scale, more images per dollar puts them on the path to profitable, scalable growth. Image: Stable Diffusion benchmark results showing a comparison of image generation time Some interesting observations from the benchmark: Deploying Stable Diffusion v1.4 on Salad Cloud Stable Diffusion v1.4 is available for 1-click deployment as a ‘Recipe’ on Salad Portal, accessible at https://portal.salad.com/. This recipe is accessible via an HTTP server, once the recipe has been deployed to Salad, you will be provided with a unique URL that can be used to access this model. In order to secure your recipe, all requests must include the Salad-Api-Key header with your individual Salad API Token that can be found in your account settings. Example API Request Parameters required prompt- Your prompt for Stable Diffusion to generate negativeprompt- Prompts for Stable Diffusion to not contain numinferencesteps- The number of steps to generate each image guidancescale- How close to the prompt your final image should be width- Width in pixels of your final image height- Height in pixels of your final image seed- The seed to generate your images from numimagesperprompt- The number of images to generate for your prompt PIPELINE- Which pipeline to use SCHEDULER- Which scheduler to use safetychecker: Enable or disable the NSFW filter on models, note some models may force this enabled anyway Example API Response Stable Diffusion XL 0.9 on consumer-grade GPUs The pace of development in the generative AI space has been tremendous. Stability.ai just announced SDXL 0.9, the most advanced development in the Stable Diffusion text-to-image suite of models. SDXL 0.9 produces massively improved image and composition detail over its predecessor. In the announcement, Stability.ai noted that SDXL 0.9 can be run on a modern consumer GPU with just 16GB RAM and a minimum of 8GB of vRAM. Chalk it up as another win for consumer-grade GPUs in the race to serve inference at scale.
GPU shortage isn’t the problem for Generative AI. GPU selection is.
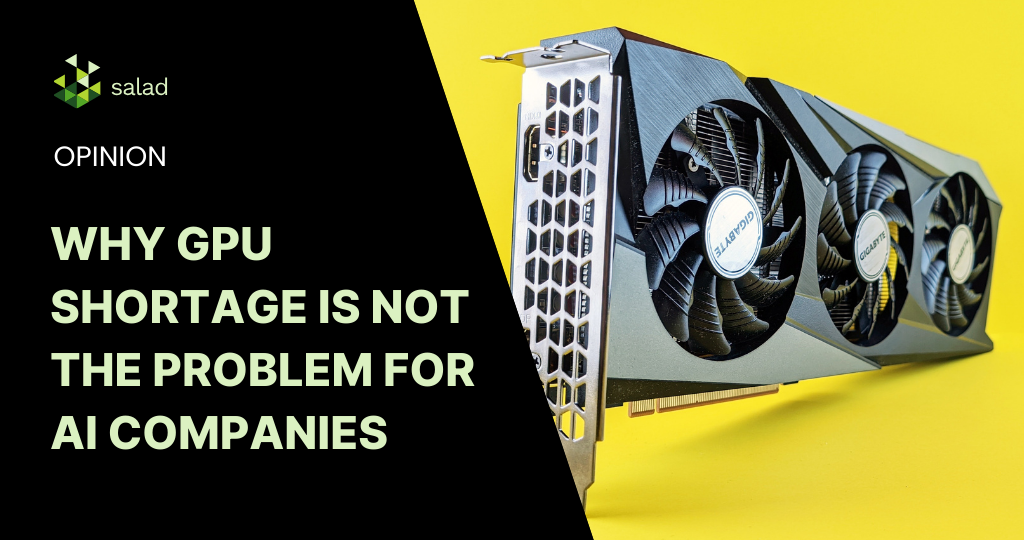
Are we truly out of GPU compute power or are we just looking in the wrong places for the wrong type of GPUs? Recently, GPU shortage has been in the news everywhere. Just take a peek at the many articles on the topic here – The Information, IT Brew, Wall Street Journal, a16z. The explosive growth of generative AI has created a mad rush and long wait-times for AI-focused GPUs. For growing AI companies serving inference at scale, shortage of such GPUs is not the real problem. Selecting the right GPU is. AI Inference Scalability and the “right-sized” GPU Today’s ‘GPU shortage’ is really a function of inefficient usage and overpaying for GPUs that don’t align with the application’s needs for at-scale AI. The marketing machines at large cloud companies and hardware manufacturers have managed to convince developers that they ABSOLUTELY NEED the newest, most powerful hardware available in order to be a successful AI company. The A100s and H100s – perfect for training and advanced models – certainly deserve the tremendous buzz for being the fastest, most advanced GPUs. But there aren’t enough of these GPUs around and when they are available, it requires pre-paying or having an existing contract. A recent article by semianalysis has two points that confirm this: Meanwhile, GPU benchmark data suggests that there are many use cases where you don’t need the newest, most powerful GPUs. Consumer-grade GPUs (RTX3090, A5000, RTX4090, etc.) not only have high availability but also deliver more inferences per dollar significantly reducing your cloud cost. Selecting the “right sized” GPU at the right stage puts generative AI companies on the path to profitable, scalable growth, lower cloud costs and immune to ‘GPU shortages’. How to Find the “Right Sized” GPU When it comes to determining the “right sized” GPU for your application, there are several factors to consider. The first step is to evaluate the needs of your application at each stage of an AI model’s lifecycle. This means taking into account the varying compute, networking and storage requirements for tasks such as data preprocessing, training, and inference. Training Models During the training stage of machine learning models, it is common for large amounts of computational resources to be required. This includes the use of high-powered graphics processing units (GPUs) which can number from hundreds to thousands. These GPUs need to be connected through lightning-fast network connections in specially designed clusters to ensure that the machine learning models receive the necessary resources to train effectively. These specially designed clusters are optimized for the specific needs of machine learning and are capable of handling the intense computational demands required during the training stage. Example: Training Stable Diffusion (Approximate Cost: $600k) Serving Models (Inference) When it comes to serving your model, scalability and throughput are particularly crucial. By carefully considering these factors, you can ensure that your infrastructure is able to accommodate the needs of your growing user base. This includes being mindful of both budgetary constraints and architectural considerations. It’s worth noting that there are many examples in which the GPU requirements for serving inference are significantly lower than those for training. Despite this, many people continue to use the same GPUs for both tasks. This can lead to inefficiencies, as the hardware may not be optimized for the unique demands of each task. By taking the time to carefully assess your infrastructure needs and make necessary adjustments, you can ensure that your system is operating as efficiently and effectively as possible. Example 1: 6X more images per dollar on consumer-grade GPUs In a recent Stable Diffusion benchmark, consumer-grade GPUs generated 4X-8X more images per dollar compared to AI-focused GPUs. Most generative AI companies in the text-to-image space will be well served using consumer-grade GPUs for serving inference at scale. The economics and availability make them a winner for this use case. Example 2: Serving Stable Diffusion SDXL In the recent announcement introducing SDXL, Stability.ai noted that SDXL 0.9 can be run on a modern consumer GPU with just 16GB RAM and a minimum of 8GB of vRAM. Serving “Right Sized” AI Inference at Scale At Salad, we understand the importance of being able to serve AI/ML inference at scale without breaking the bank. That’s why we’ve created a globally distributed network of consumer GPUs that are designed from the ground up to meet your needs. Our customers have found that turning to SaladCloud instead of relying on large cloud computing providers has allowed them to not only save up to 90% of their cloud cost, but also improve their product offerings and reduce their dev ops time. Example: Generating 9M+ images in 24 hours for only $1872 In a recent benchmark for a customer, we generated 9.2 Million Stable Diffusion images in 24 hours for just $1872 – all on Nvidia’s 3000/4000 series GPUs. That’s ~5000 images per dollar leading to significant savings for this image generation company. With SaladCloud, you won’t have to worry about costly infrastructure maintenance or unexpected downtime. If it works on your system, it works on Salad. Instead, you can focus on what really matters – serving your growing user base while remaining profitable. To see if your use case is a fit for consumer-grade GPUs, contact our team today.